A new method known as YaRN (Yet Another RoPE for Transformers) has emerged, offering the potential to extend context capabilities in large language models (LLMs) using the RoPE technique for positional coding. This approach, as detailed in a recent article, provides the means to expand context up to 64k or even 128k tokens. This innovation is particularly notable as it addresses the growing demand for models that can accommodate substantial context, such as extended texts or lengthy message histories.
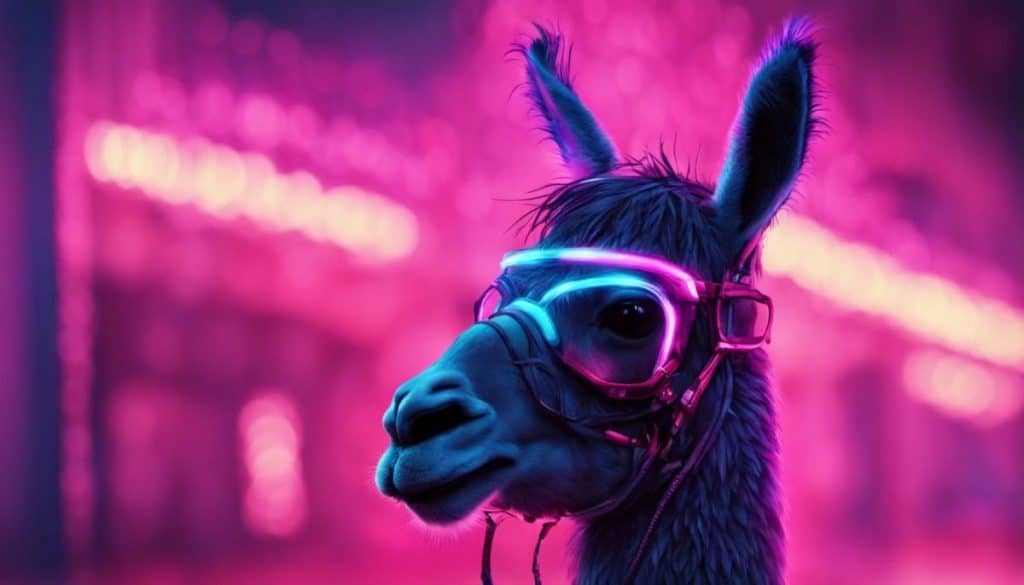
The RoPE method involves rotating vectors in space at specific angles based on their positions, and is particularly used in models like LLaMa-2. The YaRN method differs from earlier modifications, though, by adding a brand-new component: a temperature parameter that is crucial in affecting how quickly people pay attention after the softmax operation. This integration of temperature control is significant because it keeps the attention mechanisms’ original structure and prevents the need for significant changes to the existing codebase.
An intriguing aspect of YaRN’s implementation is its adaptability with existing models hosted on platforms like Hugging Face. By harnessing the power of these readily available models, researchers and practitioners can experiment with and explore the YaRN method with relative ease.
It is worth noting that YaRN, like other novel techniques, requires retraining on data containing extended contexts, albeit in a modest quantity—approximately 0.1% of the pretraining data. The primary consideration moving forward pertains to the computational resources necessary for efficiently inferring with these expanded-context models, an aspect that will play a pivotal role in the practical implementation of this innovative approach.
- YaRN opens the door to more extensive contextual understanding, offering applications that span various domains, from literature analysis to conversational AI. As the AI community continues to explore methods for enhancing model capabilities, YaRN’s nuanced approach to extending context holds the potential to provide valuable insights and improved performance in various natural language processing tasks.
- In July, Meta has released LLaMa-2-Chat models, a game-changing open-source language model with 70 billion parameters, comparable to and outperforming GPT-3.5 on certain benchmarks. The model is commercially friendly, pretrained on 2T tokens, and has strong MMLU scores. It is the first model of its size fine-tuned using RLHF, making it completely free for commercial use. LLaMa-2-Chat showcases exceptional performance on mathematical problems and is available in various sizes.
Read more about AI:
Read More: mpost.io