Video-LLaMA bringing us closer to a deeper comprehension of videos through sophisticated language processing. The acronym Video-LLaMA stands for Video-Instruction-tuned Audio-Visual Language Model, and it is based on the BLIP-2 and MiniGPT-4 models, two strong models.
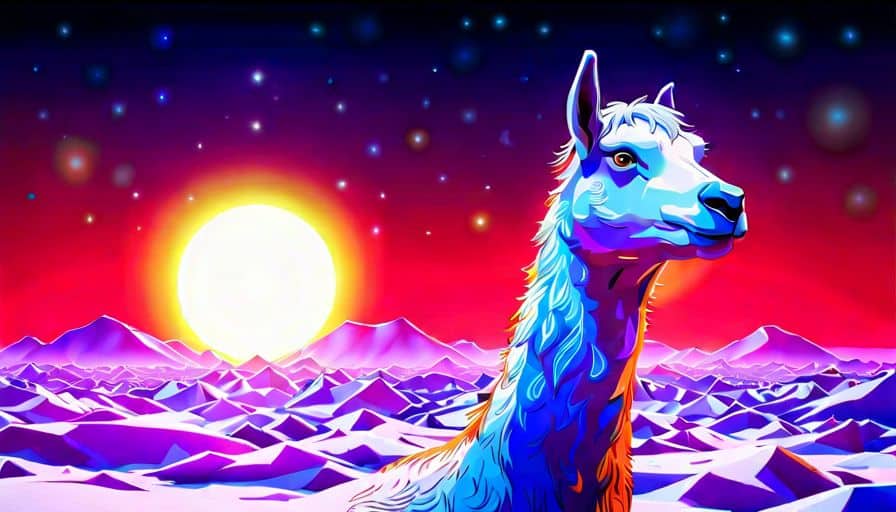
Video-LLaMA consists of two core components: the Vision-Language (VL) Branch and the Audio-Language (AL) Branch. These components work together harmoniously to process and comprehend videos by analyzing both visual and audio elements.
The VL Branch utilizes the ViT-G/14 visual encoder and the BLIP-2 Q-Former, a special type of transformer. To compute video representations, a two-layer video Q-Former and a frame embedding layer are employed. The VL Branch is trained on the Webvid-2M video caption dataset, focusing on the task of generating textual descriptions for videos. Additionally, image-text pairs from the LLaVA dataset are included during pre-training to enhance the model’s understanding of static visual concepts.
To further refine the VL Branch, a process called fine-tuning is conducted using instruction-tuning data from MiniGPT-4, LLaVA, and VideoChat. This fine-tuning phase helps Video-LLaMA adapt and specialize its video understanding capabilities based on specific instructions and contexts.
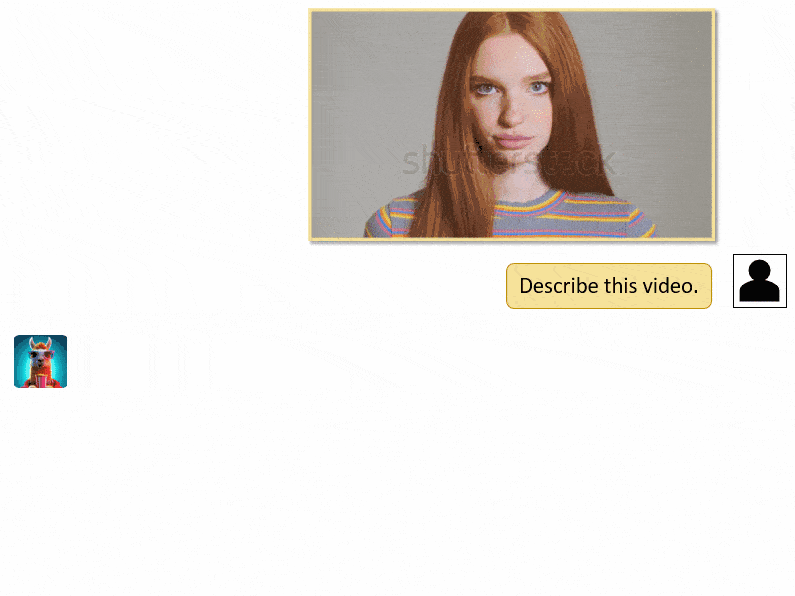
Moving on to the AL Branch, it leverages the powerful audio encoder known as ImageBind-Huge. This branch incorporates a two-layer audio Q-Former and an audio segment embedding layer to compute audio representations. As the audio encoder (ImageBind) is already aligned across multiple modalities, the AL Branch focuses solely on video and image instrucaption data to establish a connection between the output of ImageBind and the language decoder.
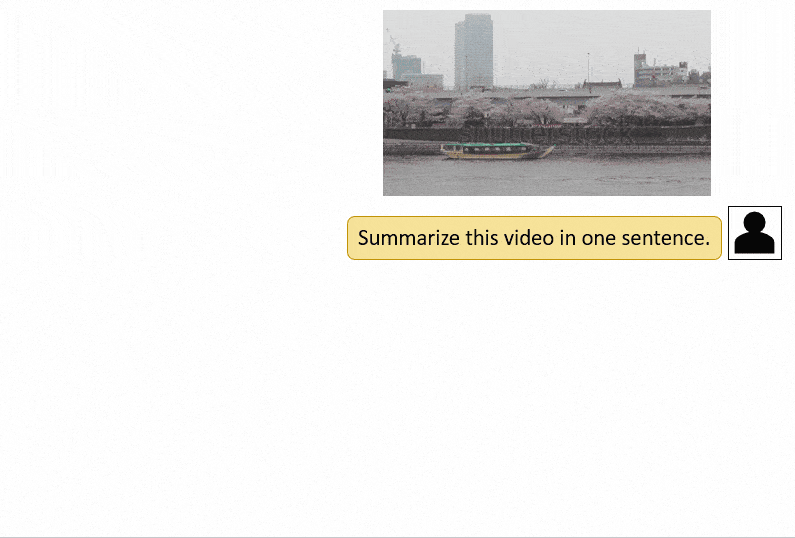
During the cross-modal training of Video-LLaMA, it is important to note that only the Video/Audio Q-Former, positional embedding layers, and linear layers are trainable. This selective training approach ensures that the model learns to effectively integrate visual, audio, and textual information while maintaining the desired architecture and alignment between modalities.
By employing state-of-the-art language processing techniques, this model opens doors to more accurate and comprehensive analysis of videos, enabling applications such as video captioning, summarization, and even video-based question answering systems. We can expect to witness remarkable advancements in fields like video recommendation, surveillance, and content moderation. Video-LLaMA paves the way for exciting possibilities in harnessing the power of audio-visual language models for a more intelligent and intuitive understanding of videos in our digital world.
Read more about AI:
Read More: mpost.io