

In Brief
MIT and Google computer scientists have unveiled StableRep, a program designed to transform descriptive written captions into accurate corresponding images using pictures generated by Stable Diffusion. This tool is geared towards enhancing the capability of neural networks to generate images based on textual descriptions. According to the researchers, synthetic images can help AI models learn visual representations more accurately compared to real photographs.
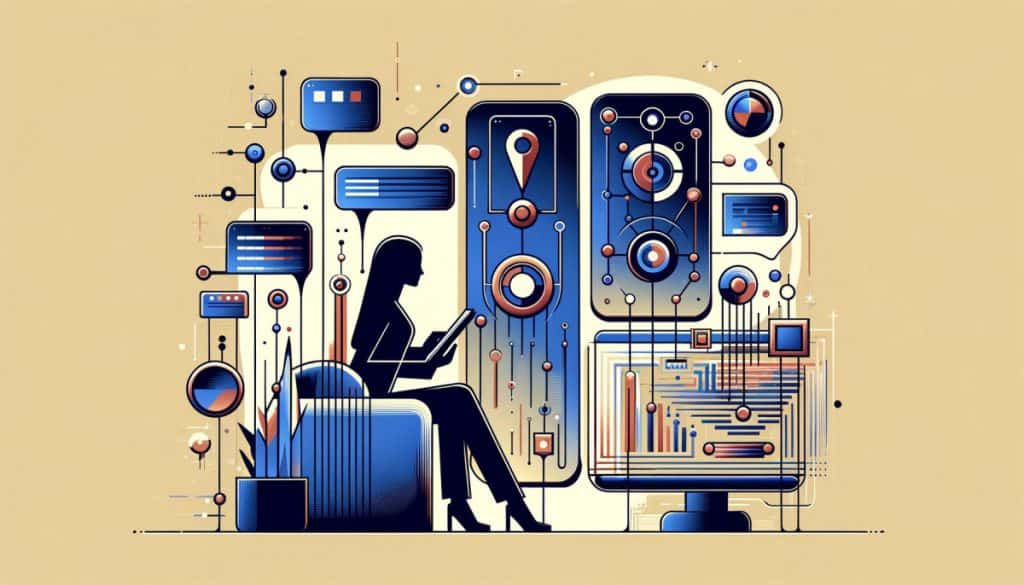
MIT and Google computer scientists have unveiled StableRep, a AI-model designed to transform descriptive written captions into accurate corresponding images using pictures generated by Stable Diffusion. This tool is geared towards enhancing the capability of neural networks to generate images based on textual descriptions.
According to the researchers, synthetic images can help AI models learn visual representations more accurately compared to real photographs.
StableRep aims to empower researchers to manage the machine learning algorithmic process by training a model on a multitude of images generated by Stable Diffusion in response to the same prompt. Thus, the model will learn a broader range of visual representations, defining which images closely align with the given prompts.
Researchers envision the emergence of an ecosystem of AI models, some of which will be trained on either real or synthetic data. Presently, efforts are focused on teaching the model to learn more about high-level concepts through contextual understanding and variability, instead of simply feeding it data.
StableRep Will Help AI Developers and Engines
At the core of text-to-image models lies their capability to link objects with words. When presented with an input text prompt, these models should generate an image that closely matches the provided description. To achieve this, they must acquire an understanding of the visual representations of real-world objects.
According to a recent pre-print paper on arXiv, StableRep outperforms SimCLR and CLIP in terms of learned representations using the same set of text prompts and corresponding real images on large-scale datasets, solely relying on synthetic images.
The paper continues, “When we further introduce language supervision, StableRep trained with 20 million synthetic images achieves better accuracy than CLIP trained with 50 million real images.”
SimCLR and CLIP are machine-learning algorithms employed for generating images from text prompts.
This innovative approach enables AI developers to train neural networks with fewer synthetic images than real ones while achieving better results. The emergence of StableRep-like methods suggests a future where text-to-image models could be trained predominantly on synthetic data, reducing dependence on real images and supporting AI engines when faced with limitations in available online resources.
Disclaimer
In line with the Trust Project guidelines, please note that the information provided on this page is not intended to be and should not be interpreted as legal, tax, investment, financial, or any other form of advice. It is important to only invest what you can afford to lose and to seek independent financial advice if you have any doubts. For further information, we suggest referring to the terms and conditions as well as the help and support pages provided by the issuer or advertiser. MetaversePost is committed to accurate, unbiased reporting, but market conditions are subject to change without notice.
About The Author
Alisa is a reporter for the Metaverse Post. She focuses on investments, AI, metaverse, and everything related to Web3. Alisa has a degree in Business of Art and expertise in Art & Tech. She has developed her passion for journalism through writing for VCs, notable crypto projects, and engagement with scientific writing.

Alisa Davidson

Alisa is a reporter for the Metaverse Post. She focuses on investments, AI, metaverse, and everything related to Web3. Alisa has a degree in Business of Art and expertise in Art & Tech. She has developed her passion for journalism through writing for VCs, notable crypto projects, and engagement with scientific writing.
Read More: mpost.io