As the 60th presidential election in the United States approaches, the role of the internet and social networks in shaping political discourse is under scrutiny, especially in the aftermath of the Cambridge Analytica scandal. A significant question arises: What will the digital landscape look like during the upcoming elections and new achievements in AI?
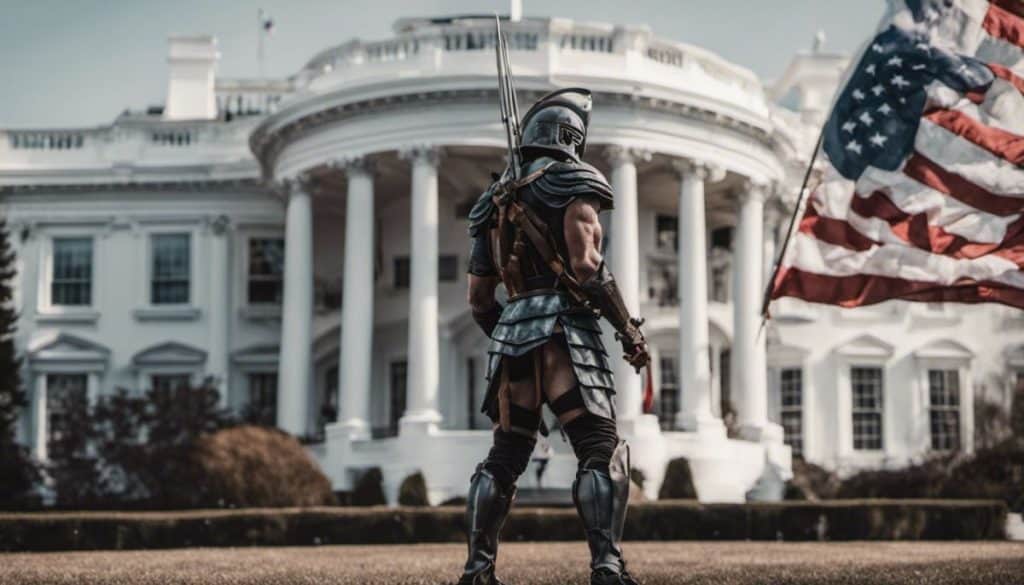
During recent Senate hearings, Senator Josh Hawley of Missouri raised this critical issue in the context of language models. He referred to an article titled “Language Models Trained on Media Diets Can Predict Public Opinion” authored by researchers from MIT and Stanford. This research explores the potential of using neural networks to predict public opinion based on news articles, a concept that could significantly impact political campaigns.
The article describes a methodology where language models are initially trained on specific sets of news articles to predict missing words within a given context, similar to BERT models. The subsequent step involves assigning a score, denoted as “s,” to evaluate the model’s performance. Here’s an overview of the process:
- A thesis statement is formulated, for instance, “Request for the closure of most businesses, except grocery stores and pharmacies, in order to combat the coronavirus outbreak.”
- Notably, there is a blank in the thesis. Language models are utilized to estimate the probabilities of completing this gap with specific words.
- The likelihood of various words, such as “necessary” or “unnecessarily,” is assessed.
- This probability is normalized relative to a base undertrained model, which gauges the frequency of a word occurring in a given context independently. The resulting fraction represents the score “s,” which characterizes the new information introduced by the dataset from the media concerning existing knowledge.
The model accounts for the level of engagement of a particular group of individuals with news on a specific topic. This additional layer enhances prediction quality, as measured by the correlation between the model’s predictions and people’s opinions regarding the original thesis.
The secret lies in the fact that theses and news were categorized based on their dates. By studying the news related to the initial months of the coronavirus outbreak, it became possible to anticipate people’s reactions to proposed measures and changes.
The metrics may not appear impressive, and the authors themselves emphasize that their findings do not imply that AI can completely replace human involvement in the process, or models can replace human surveys. Instead, these AI tools serve as aids in summarizing vast amounts of data and identifying potentially fruitful areas for further exploration.
Interestingly, a senator arrived at a different conclusion, expressing concern about the models performing too well and the potential dangers associated with this. There is some validity to this perspective, considering that the article showcases rather basic models, and future iterations like GPT-4 could potentially offer significant improvements.
The Growing Challenge of AI-Driven Social Network Manipulation
In recent discussions, the conversation steered away from the impending presidential elections and towards the concerning topic of employing Language Model Models (LLMs), even on a localized scale, to fabricate and populate fake accounts across social networks. This discussion underscores the potential for automating troll factories with an emphasis on propaganda and ideological influence.
While this may not appear groundbreaking considering the technology already in use, the difference lies in scale. LLMs can be employed continuously, limited only by the allocated GPU budget. Furthermore, to maintain conversations and threads, additional, less advanced bots can join discussions and respond. Their effectiveness in persuading users is dubious. Will a well-crafted bot genuinely change someone’s political stance, prompting them to think, “What have these Democrats done? I should vote for the Republicans”?
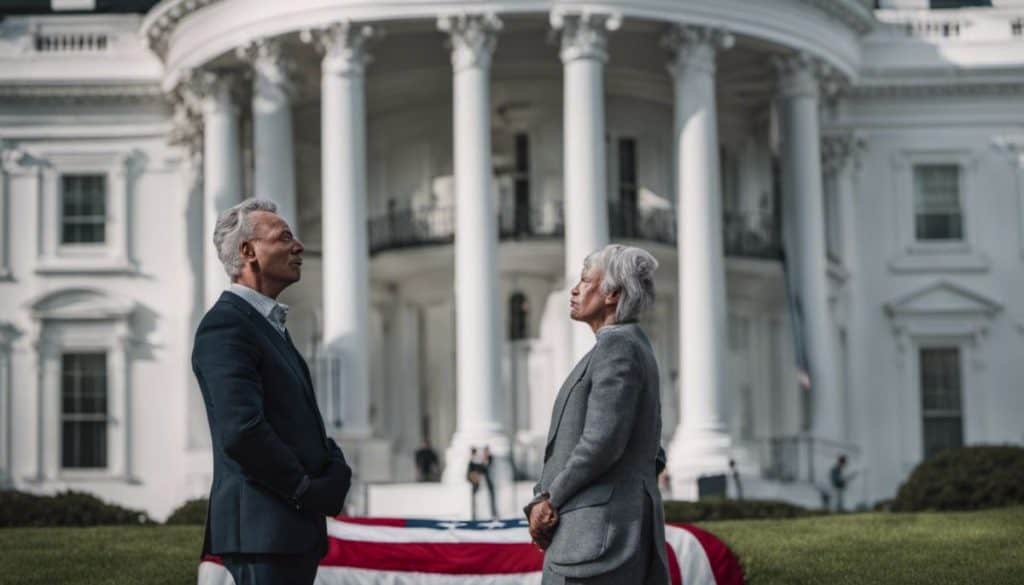
Attempting to assign a troll employee to each online user for systematic persuasion is impractical, reminiscent of the joke “half sits, half stands.” In contrast, a bot empowered with advanced neural networks remains tireless, capable of engaging with tens of millions of individuals simultaneously.
A potential countermeasure involves prepping social media accounts by simulating human-like behavior. Bots can mimic genuine users by discussing personal experiences and posting diverse content while maintaining an appearance of normalcy.
While this may not be a pressing issue in 2024, it is increasingly likely to become a significant challenge by 2028. Addressing this problem poses a complex dilemma. Should social networks be disabled during the election season? Unfeasible. Educating the public not to unquestionably trust online content? Impractical. Losing elections due to manipulation? Undesirable.
An alternative could involve advanced content moderation. The shortage of human moderators and the limited effectiveness of existing text detection models, even those from OpenAI, cast doubt on the viability of this solution.
OpenAI’s GPT-4 Updates Content Moderation with Rapid Rule Adaptation
OpenAI, under the guidance of Lilian Weng, has recently introduced a project called “Using GPT-4 for Content Moderation.” This accelerates the process of updating content moderation rules, reducing the timeline from months to mere hours. GPT-4 exhibits an exceptional ability to comprehend rules and subtleties within comprehensive content guidelines, instantly adapting to any revisions, thereby ensuring more consistent content assessment.
This sophisticated content moderation system is ingeniously straightforward, as demonstrated in an accompanying GIF. What sets it apart is GPT-4’s remarkable proficiency in understanding written text, a feat not universally mastered even by humans.
Here’s how it operates:
- After drafting moderation guidelines or instructions, experts select a limited dataset containing instances of violations and assign corresponding labels in adherence to the violation policy.
- GPT-4 subsequently comprehends the rule set and labels the data without access to the responses.
- In cases of disparities between GPT-4 responses and human judgments, experts can solicit clarifications from GPT-4, analyze ambiguities within the instruction definitions, and dispel any confusion through additional clarification, marked with blue step text in the GIF.
This iterative process of steps 2 and 3 can be repeated until the algorithm’s performance meets the desired standard. For large-scale applications, GPT-4 predictions can be employed to train a significantly smaller model, which can deliver comparable quality.
OpenAI has disclosed metrics for assessing 12 distinct types of violations. On average, the model outperforms standard content moderators, but it still lags behind the expertise of seasoned and well-trained human moderators. Nevertheless, one compelling aspect is its cost-effectiveness.
It’s worth noting that machine learning models have been utilized in auto-moderation for several years. The introduction of GPT-4 is poised to usher in new innovations, particularly in the realm of politics and elections. There is even speculation that OpenAI could become the exclusive provider of the officially sanctioned TrueModerationAPI™ by the White House, especially in light of their recent partnership endeavors. The future holds exciting possibilities in this domain.
Read more about AI:
Read More: mpost.io